sequence model coursera week 4 quiz answers
Quiz - Transformers
1. A Transformer Network processes sentences from left to right, one word at a time.
- True
- False
2. Transformer Network methodology is taken from: (Check all that apply)
- Convolutional Neural Network style of processing.
- None of these.
- Attention mechanism.
- Convolutional Neural Network style of architecture.
3. What are the key inputs to computing the attention value for each word?
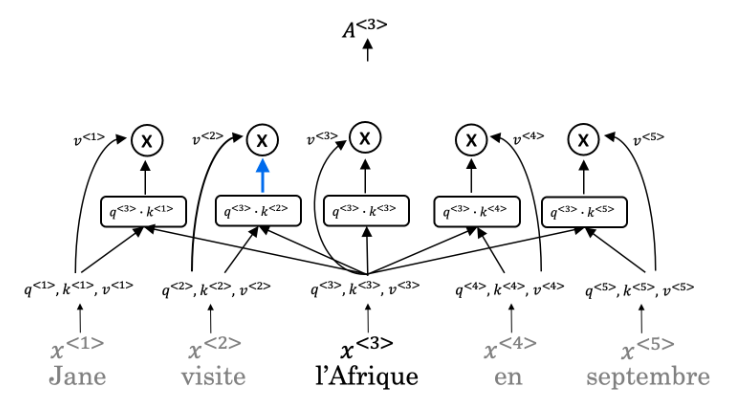
- The key inputs to computing the attention value for each word are called the query, key, and value.
- The key inputs to computing the attention value for each word are called the query, knowledge, and vector.
- The key inputs to computing the attention value for each word are called the quotation, key, and vector.
- The key inputs to computing the attention value for each word are called the quotation, knowledge, and value.
5. Are the following statements true regarding Query (Q), Key (K) and Value (V)?
Q = interesting questions about the words in a sentence
K = qualities of words given a Q
V = specific representations of words given a Q
- True
- False
6. What does i i represent in this multi-head attention computation?

- The computed attention weight matrix associated with the order of the words in a sentence
- The computed attention weight matrix associated with specific representations of words given a Q
- The computed attention weight matrix associated with the ith “head” (sequence)
- The computed attention weight matrix associated with the ith “word” in a sentence.
7. Following is the architecture within a Transformer Network (without displaying positional encoding and output layers(s)).

What is generated from the output of the Decoder’s first block of Multi-Head Attention?
- K
- Q
- V
8. Following is the architecture within a Transformer Network (without displaying positional encoding and output layers(s)).

The output of the decoder block contains a softmax layer followed by a linear layer to predict the next word one word at a time.
- False
- True
9. Which of the following statements is true about positional encoding? Select all that apply.
- Positional encoding provides extra information to our model.
- Positional encoding is used in the transformer network and the attention model.
- Positional encoding uses a combination of sine and cosine equations.
- Positional encoding is important because position and word order are essential in sentence construction of any language.
10. Which of these is a good criterion for a good positionial encoding algorithm?
- Distance between any two time-steps should be inconsistent for all sentence lengths.
- It should output a common encoding for each time-step (word’s position in a sentence).
- The algorithm should be able to generalize to longer sentences.
- It must be nondeterministic.